
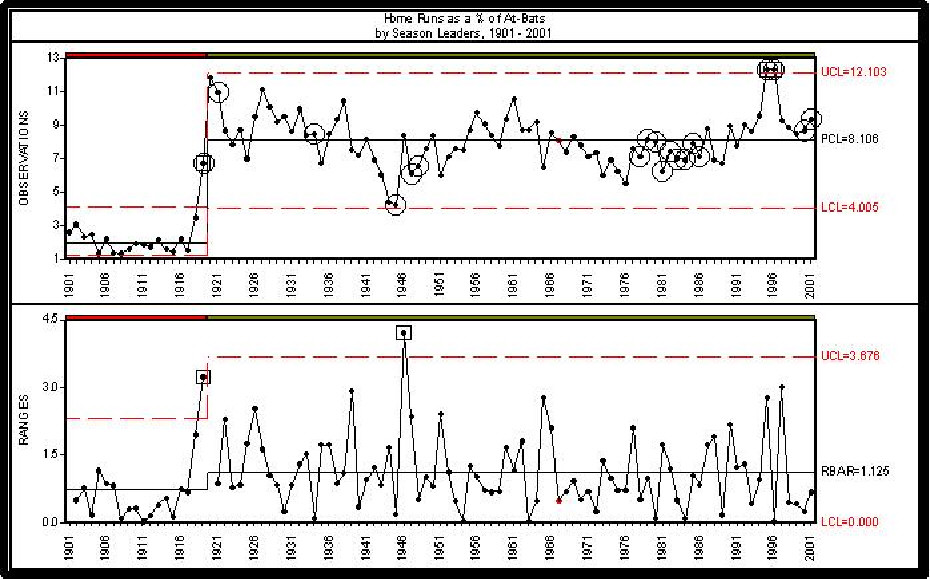
Data Scrubbing: Raw data may be collected in several different formats, with lots of junk values and clutter.In this example, data might be collected from a variety of sources like DMV or police accident reports, insurance claims and hospitalization details. Data Collection: Collect data that is useful to answer the questions.For example, do red sports cars get into accidents more often than others? Figure out which data analysis tools will get the best result for your question. Posing Questions: Figure out the questions you would like answered by the data.To get the best results out of the data, the objectives should be crystal clear. Defining Objectives: Start by outlining some clearly defined objectives.Next, using econometric models, I analyze whether these “Moneyball” teams and GMs, have enjoyed a pay-performance advantage over the rest of MLB, and whether this advantage persists after the information becomes public.Concept of big data processing and storage: cloud to databaseĭata analysis is a big subject and can include some of these steps: First, I identify “Moneyball” teams and executives, i.e., those who rely on baseball data analytics, and track their pay/performance over time. The second research question is whether this strategic advantage can be sustained once this proprietary organizational knowledge becomes public.

The first research question that this study examines is whether the organizational knowledge related to baseball data analytics has provided any advantage in the competitive Major League Baseball (MLB) marketplace. Moreover, it changed managerial vocabulary, as the term “Moneyballing” now commonly describes organizations that use data analytics. This resurgence is attributable to Moneyball, which exposed readers to the use of advanced baseball analytics by the Oakland Athletics, and how it has resulted in improved player selection and game management.

The use of data analytics has enjoyed resurgence over the last two decades in professional sports, businesses, and the government.
